Unlocking Smarter Trades: AI Sentiment Models Explained
By SYGNAL
August 21, 2024
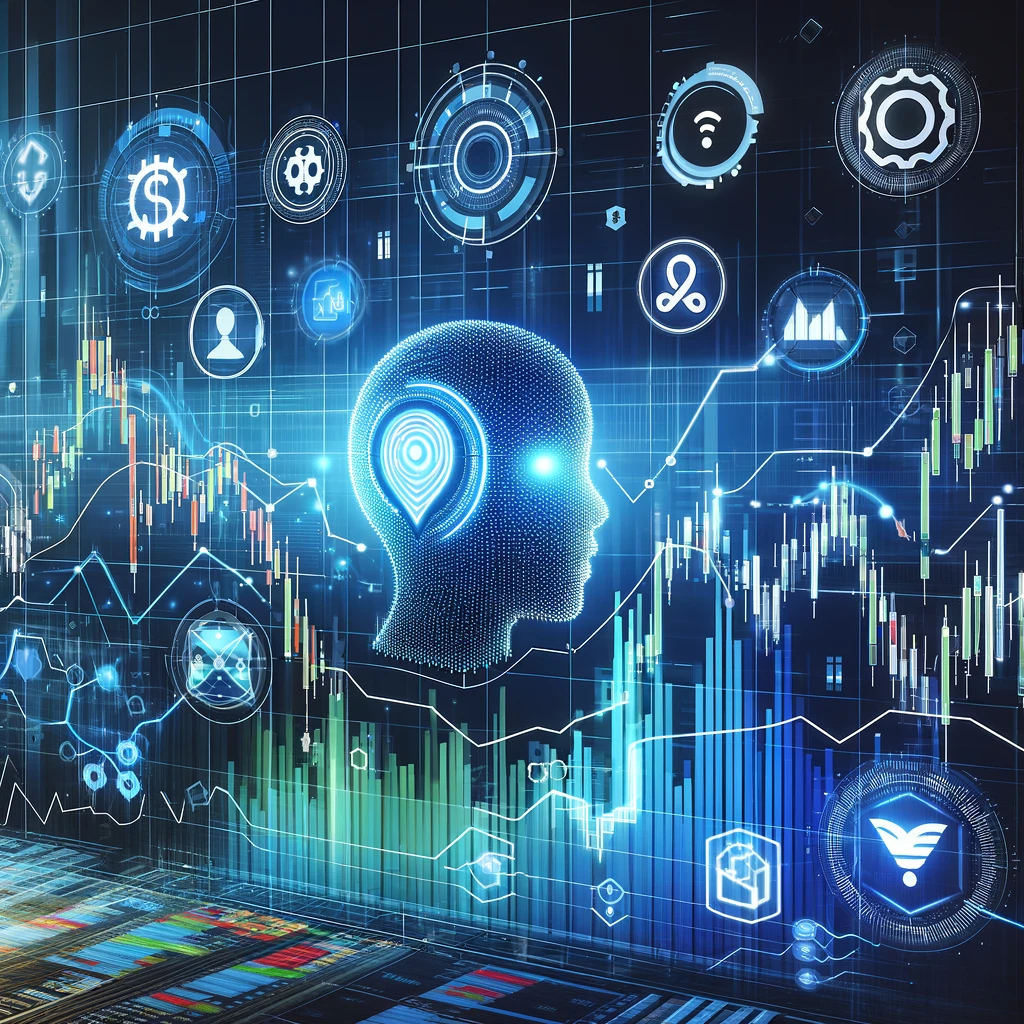
Dive into the world of AI-driven trading. Learn how sentiment models can provide deeper insights into market trends by analyzing data from social media, news, and more. See how these tools can help you make better trading decisions and enhance your strategy.
Unlocking AI Sentiment Trading
Integrating AI into trading strategies is not just about keeping up with technology; it's about gaining a competitive edge.
In the ever-evolving world of trading, staying ahead of market trends is crucial. Enter AI sentiment trading models, a sophisticated blend of Natural Language Processing (NLP), Artificial Intelligence (AI), and Machine Learning (ML) that are transforming how traders analyze and act on market sentiment. These advanced tools sift through vast amounts of data from social media, news articles, and other sources, providing traders with actionable insights that traditional methods might miss.
Imagine AI as a diligent, tireless assistant who's always on the lookout for the latest market buzz, whether it's a tweet about Bitcoin or a breaking news article on Ethereum. AI can gauge the market's mood and predict potential price movements by processing this information. This gives traders a significant edge, helping them make more intelligent, informed decisions.
Integrating AI into trading strategies is not just about keeping up with technology; it's about gaining a competitive edge. By understanding and predicting market movements through sentiment analysis, traders can make more informed and timely decisions, optimize their strategies, and maximize returns.
In this article, we'll explore the magic behind AI sentiment trading, delve into its technological foundations, examine its effectiveness, and navigate the challenges it presents. We'll also highlight how SYGNAL's innovative AI-driven strategies, like Fusion AI and AltcoinWave AI, harness the power of sentiment analysis and blockchain-based auditing to set new standards in transparency and reliability. Whether you're a seasoned trader or just starting, understanding these cutting-edge tools can help you stay ahead in the fast-paced trading world.
The Magic Behind AI Sentiment
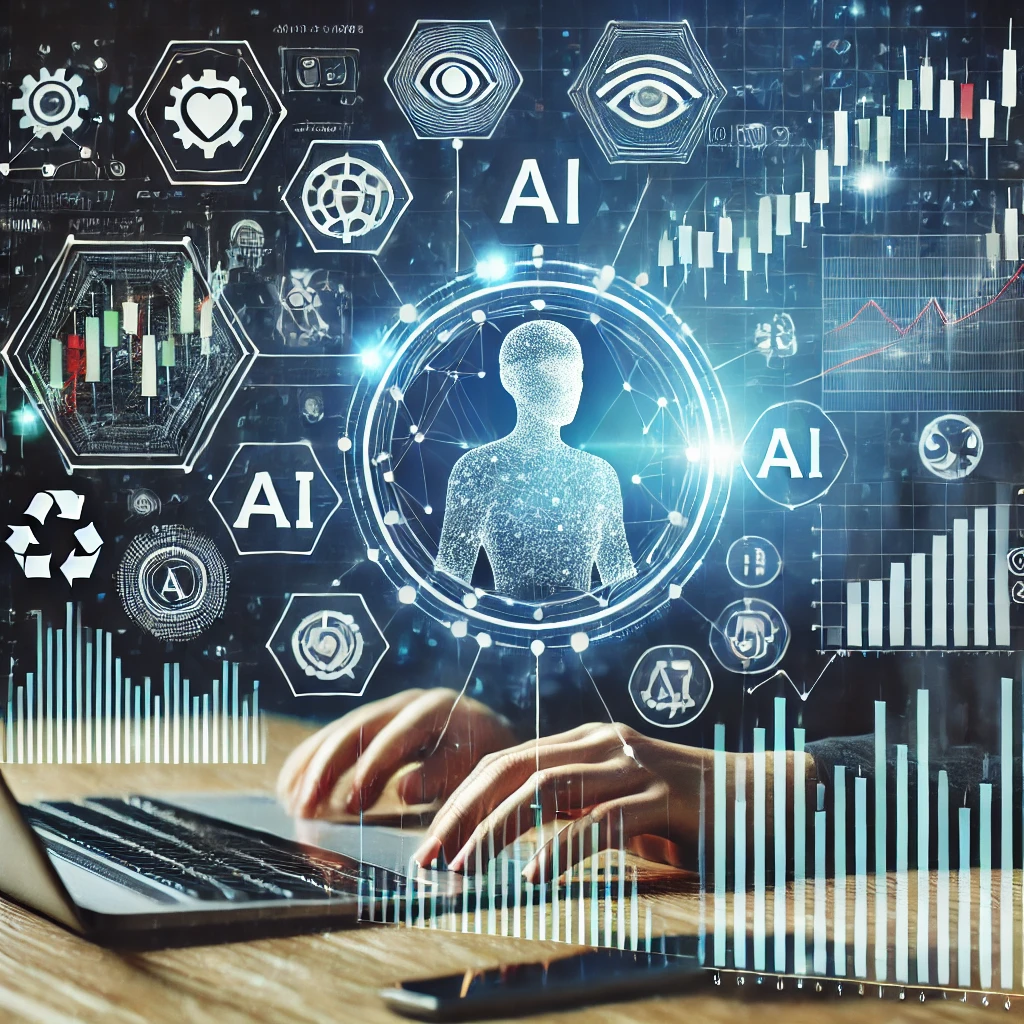
AI sentiment trading models might sound complex, but at their core, they're designed to help traders make more intelligent decisions by analyzing market sentiment. These models leverage three key technologies: Natural Language Processing (NLP), Artificial Intelligence (AI), and Machine Learning (ML).
Natural Language Processing (NLP): Think of NLP as a sophisticated language interpreter for machines. It helps computers understand and process human language, whether a tweet about Bitcoin or a financial news article. By breaking down the text, NLP can identify sentiments, such as whether the tone is positive, negative, or neutral.
Artificial Intelligence (AI): AI is the brains behind the operation. It learns from vast amounts of data, recognizing patterns and making predictions based on the sentiments extracted by NLP. It's like having a highly knowledgeable assistant who is always on top of market moods.
Machine Learning (ML): ML takes it further by constantly improving the AI's accuracy. ML algorithms learn and adapt as more data flows in, making the AI better at predicting market movements over time.
Why AI Matters in Trading
AI sentiment trading models are powerful because they provide insights that traditional analysis might miss. Here's how they work and why they're beneficial:
Data Collection: They gather data from various sources like social media, news outlets, and financial reports.
Sentiment Analysis: NLP processes this data to gauge market sentiment.
Predictive Modeling: AI uses these sentiments to predict market trends.
Execution of Trades: Automated systems can execute trades based on these predictions.
AI sentiment models help traders make informed decisions by processing this data, turning raw sentiment into actionable insights. The result? Traders can stay ahead of the curve, leveraging the market's collective mood to make more intelligent trades.
The Tech Wizardry of Sentiment Analysis
Inside the AI Engine
The fusion of NLP, AI, and ML enables these models to sift through mountains of data, transforming raw information into valuable insights:
NLP deciphers language nuances, ensuring the AI understands the context and meaning behind words. It's like having a sophisticated translator that can detect whether a news article is optimistic about Bitcoin or if a tweet is pessimistic about Ethereum.
AI applies learned patterns to predict future market movements based on current sentiment. Imagine having an astute analyst who never sleeps, constantly evaluating market moods.
ML continuously refines these predictions, enhancing accuracy with every piece of new data. This is like having a diligent assistant who gets smarter with every trade, continually improving their market predictions.
From Data to Decisions
Sentiment analysis tools scan various data sources:
Social Media: Platforms like X and Facebook are goldmines for sentiment data, reflecting real-time public opinion.
News Articles: Headlines and articles provide insights into how events are perceived by the market.
Blogs and Forums: These sources offer detailed discussions and opinions that can be crucial for sentiment analysis.
Sentiment Analysis Process
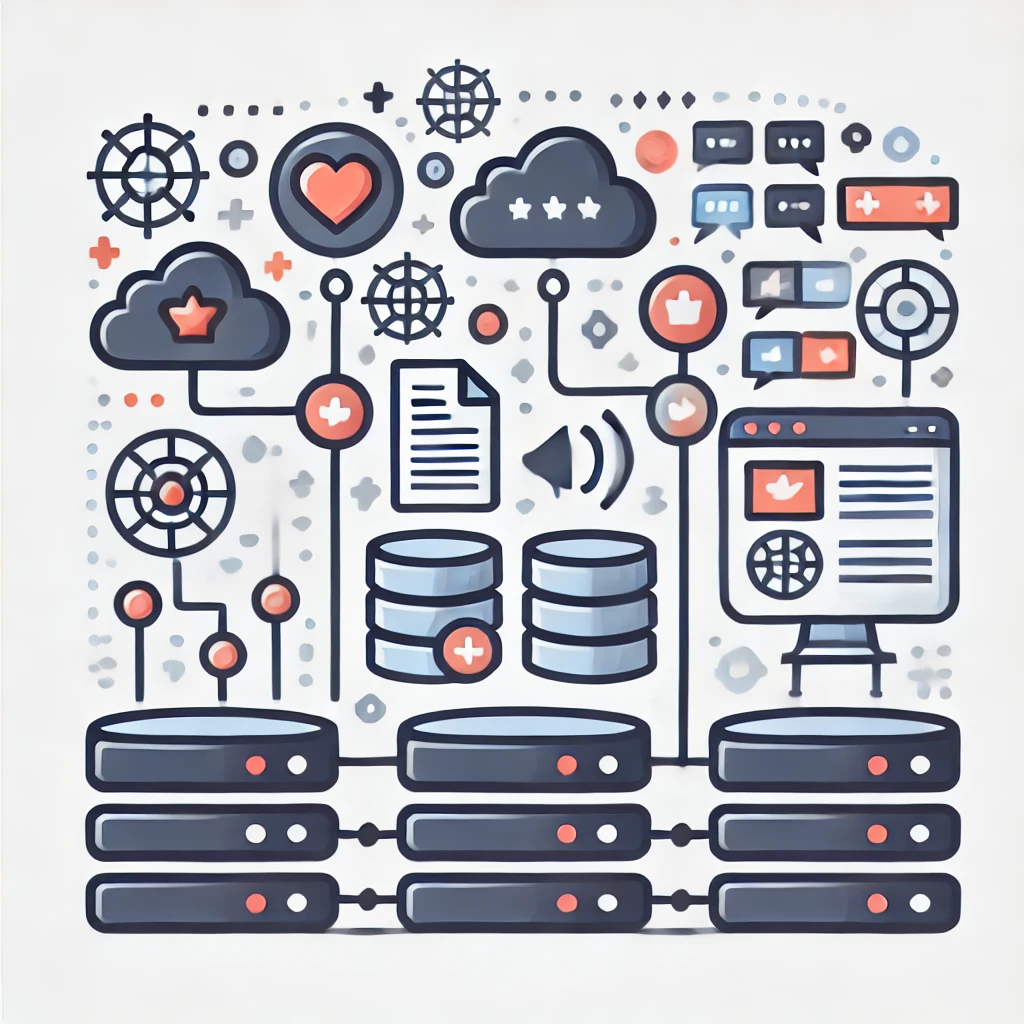
Data Collection
Gathering data from social media, news articles, blogs, and forums.
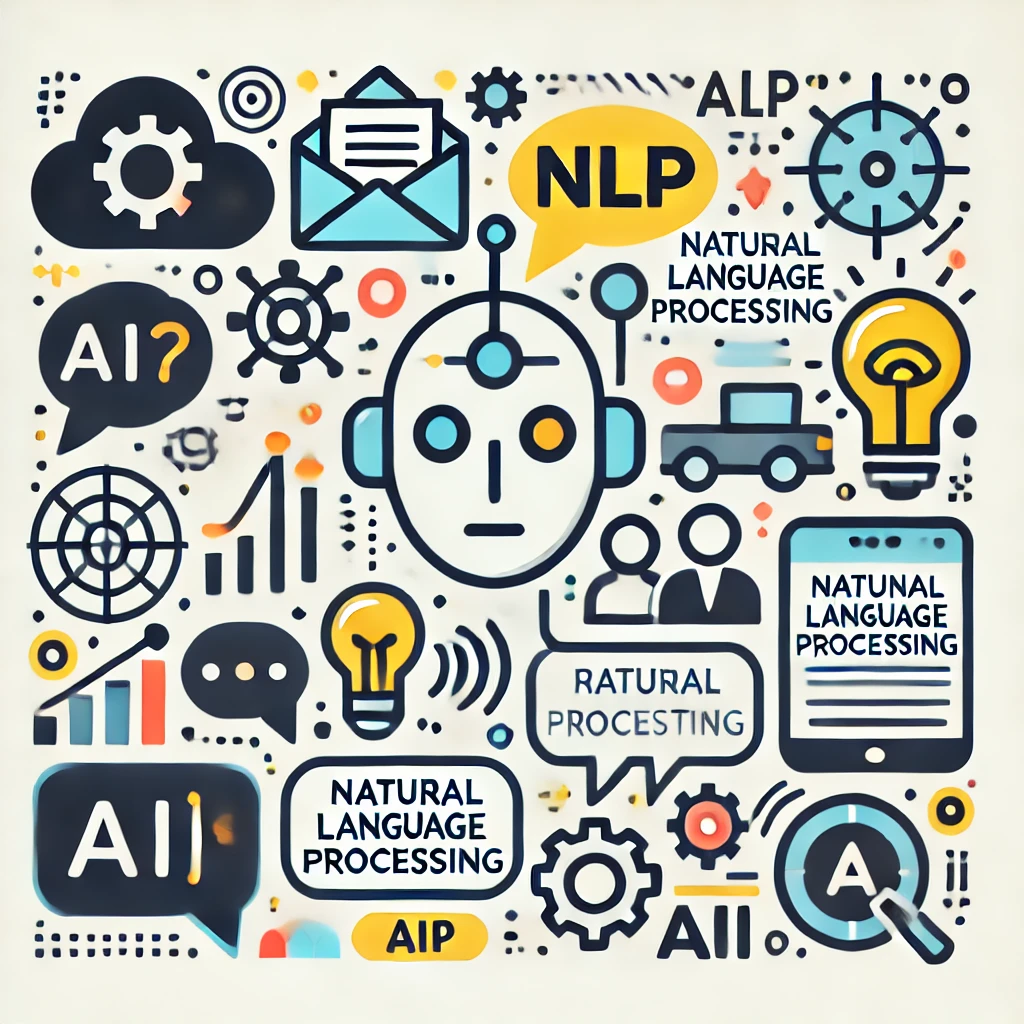
NLP
Deciphering language nuances to understand context and meaning.
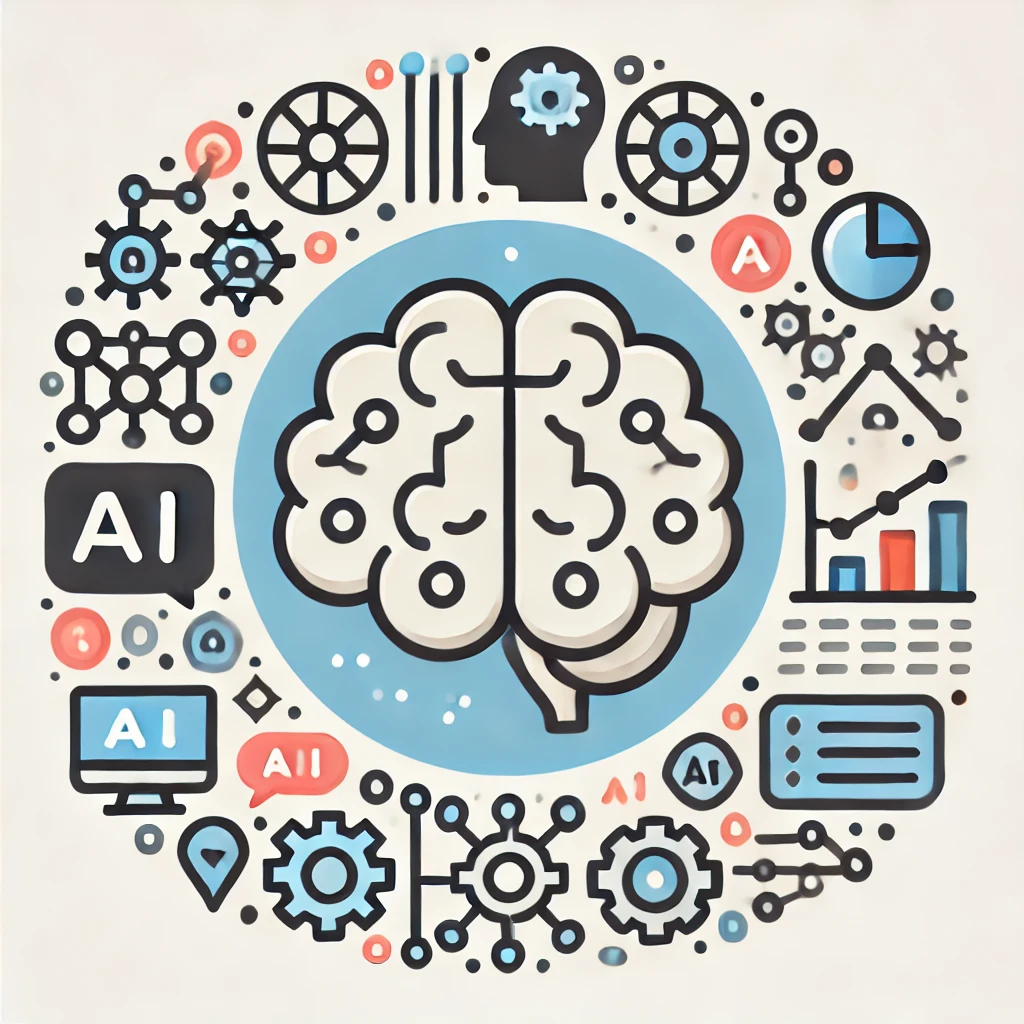
AI
Applying learned patterns to predict future market movements.
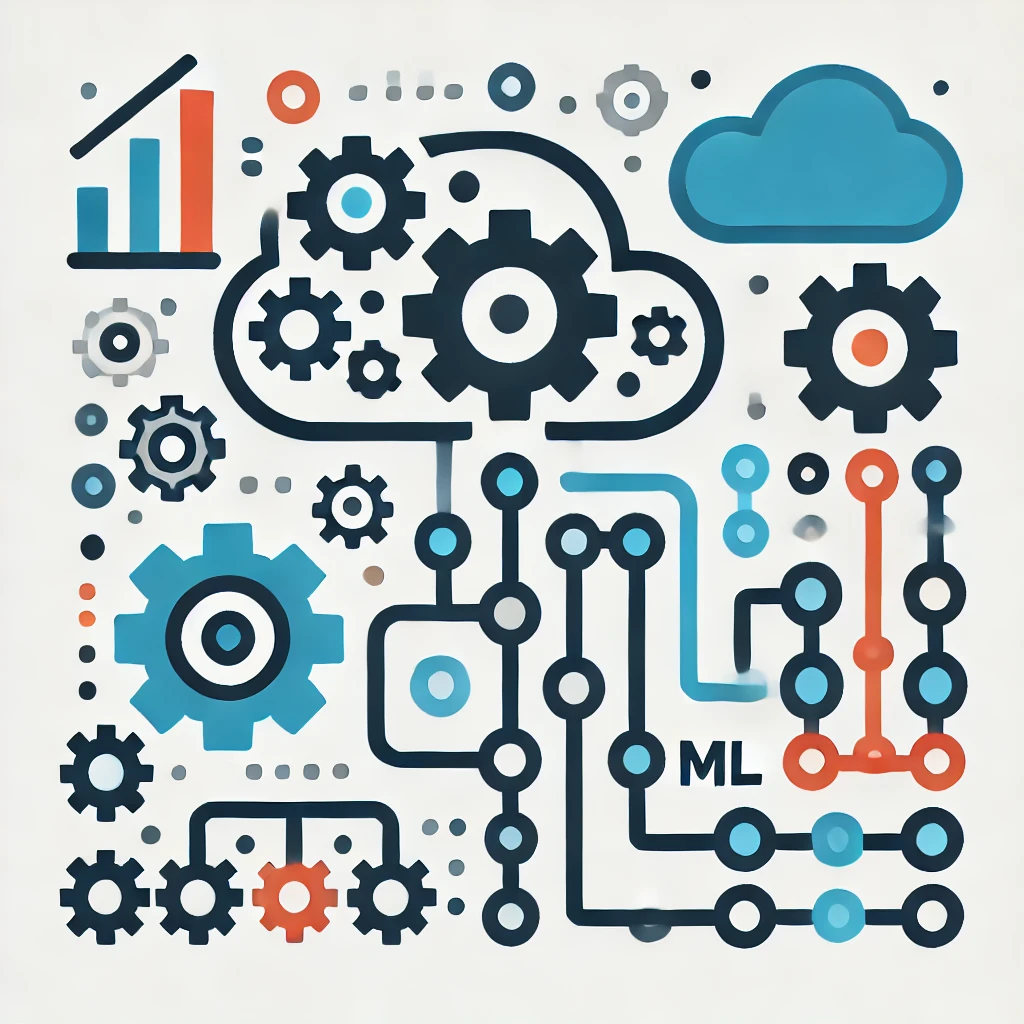
ML
Refining predictions by continuously learning from new data.
AI sentiment models help traders make informed decisions by processing this data, turning raw sentiment into actionable insights. The result? Traders can stay ahead of the curve, leveraging the market's collective mood to make more intelligent trades.
How Effective Are These Models?
AI Accuracy in Action
AI sentiment trading models have proven highly effective in predicting market movements, offering traders a competitive edge. Here's why they work:
Sentiment Indicators: These models analyze how sentiment correlates with historical price data, identifying patterns often preceding market movements. For example, a surge in positive sentiment about a particular cryptocurrency on social media can indicate a potential price increase, allowing traders to act early.
Predictive Power: AI models enhance the accuracy of predictions by integrating sentiment analysis with traditional trading strategies. The continuous learning capabilities of AI and ML mean that these models become more precise over time, adapting to new data and market conditions.
Real-World Success Stories
AI sentiment trading models are not just theoretical concepts; they have been successfully implemented in real-world trading strategies. Here are a couple of notable examples:
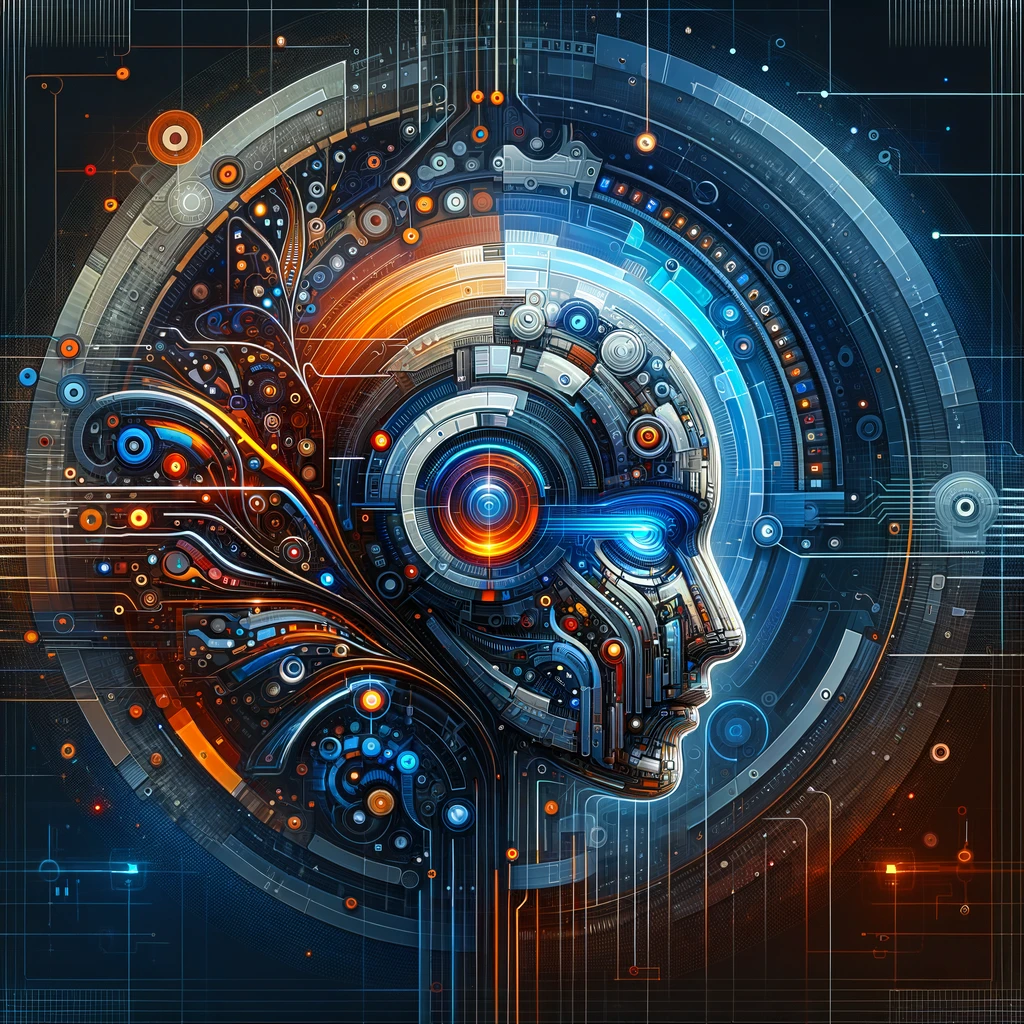
This strategy combines traditional trading methodologies with advanced AI-driven sentiment analysis. It provides daily actionable insights, helping traders optimize returns in the volatile crypto market. By merging sentiment trends with price data and technical indicators, Fusion AI offers a multidimensional approach to trading.
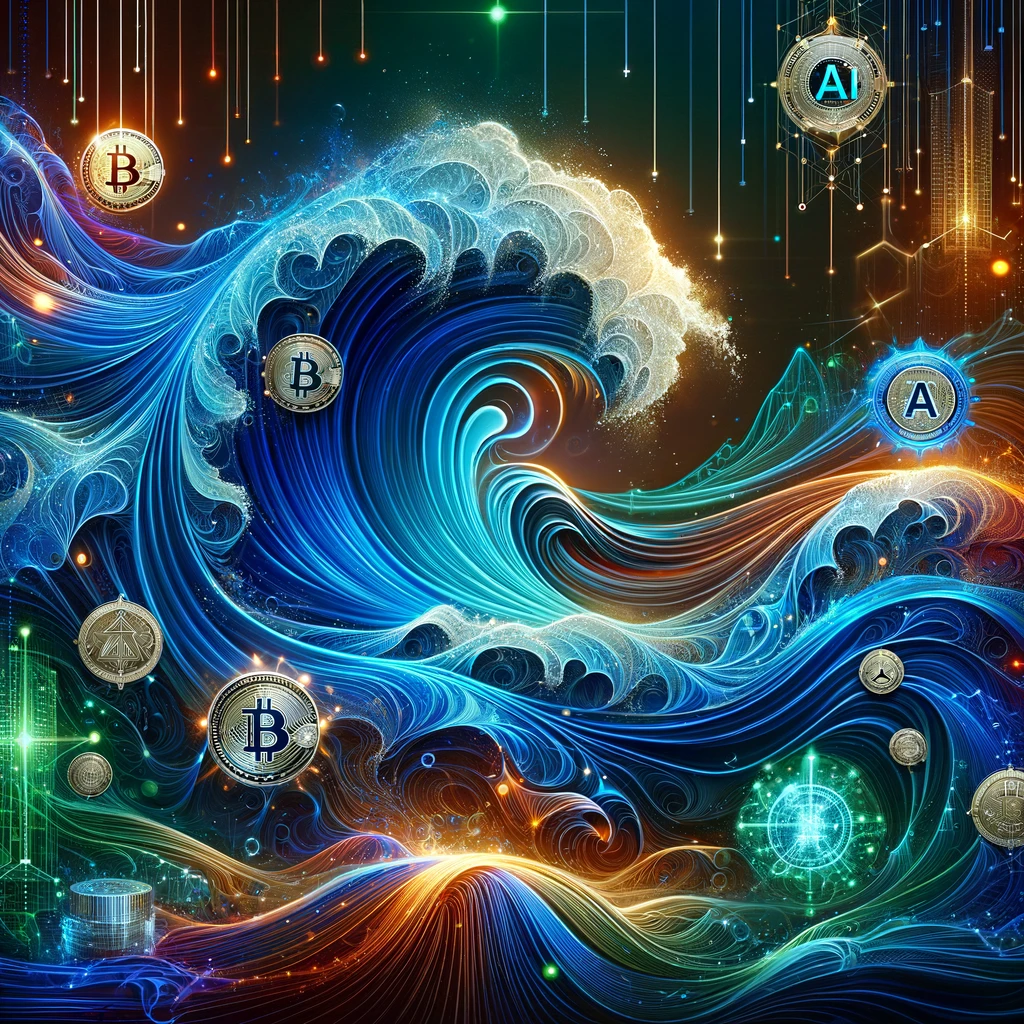
This strategy leverages proprietary AI to manage top altcoins. This strategy aims to outperform Bitcoin and maximize traders' returns by incorporating sentiment analysis and trend-following techniques.
The effectiveness of these models is evident from their performance metrics, demonstrating significant returns and improved risk management for traders who use them.
Taming the AI Beast
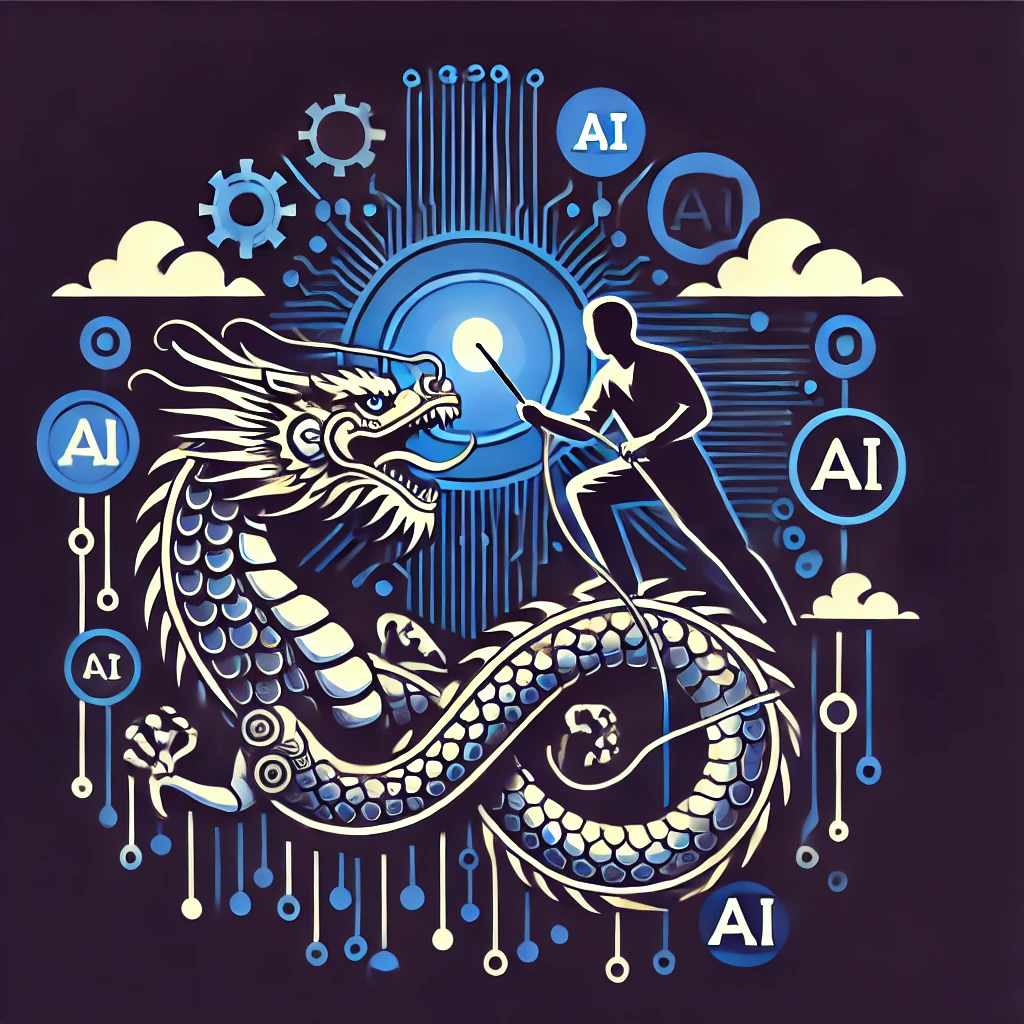
While AI sentiment trading models offer impressive capabilities, they are not without their challenges. Here are some of the critical limitations and how traders can navigate them:
Handling Ambiguous Data: AI systems often struggle with ambiguous language that can be interpreted in multiple ways. Sarcasm, slang, and context-dependent phrases can confuse even the most advanced models. This can lead to incorrect sentiment analysis, potentially causing misleading signals. Traders should be aware of this limitation and use AI sentiment analysis as one of several tools in their decision-making process.
Rapid Sentiment Shifts: The financial markets can be highly volatile, with investor sentiment capable of shifting dramatically within short periods. AI models may not always update quickly enough to catch these rapid changes, resulting in outdated or irrelevant data being used for trading decisions. Therefore, combining AI insights with real-time monitoring and human judgment is crucial.
Ethical and Regulatory Considerations: Using AI in trading raises several ethical and regulatory issues. Privacy concerns arise from the extensive data collection needed for sentiment analysis. Additionally, proprietary algorithms can create transparency issues, making it difficult for regulators and investors to understand how decisions are made. Ensuring compliance with regulations and maintaining ethical standards is essential for the sustainable use of AI in trading.
Navigating these challenges requires a balanced approach that leverages AI's strengths while being mindful of its limitations. By combining AI insights with traditional analysis and human oversight, traders can optimize their strategies and manage risks effectively.
How SYGNAL Uses Sentiment-Based AI
SYGNAL's AI Powerhouses
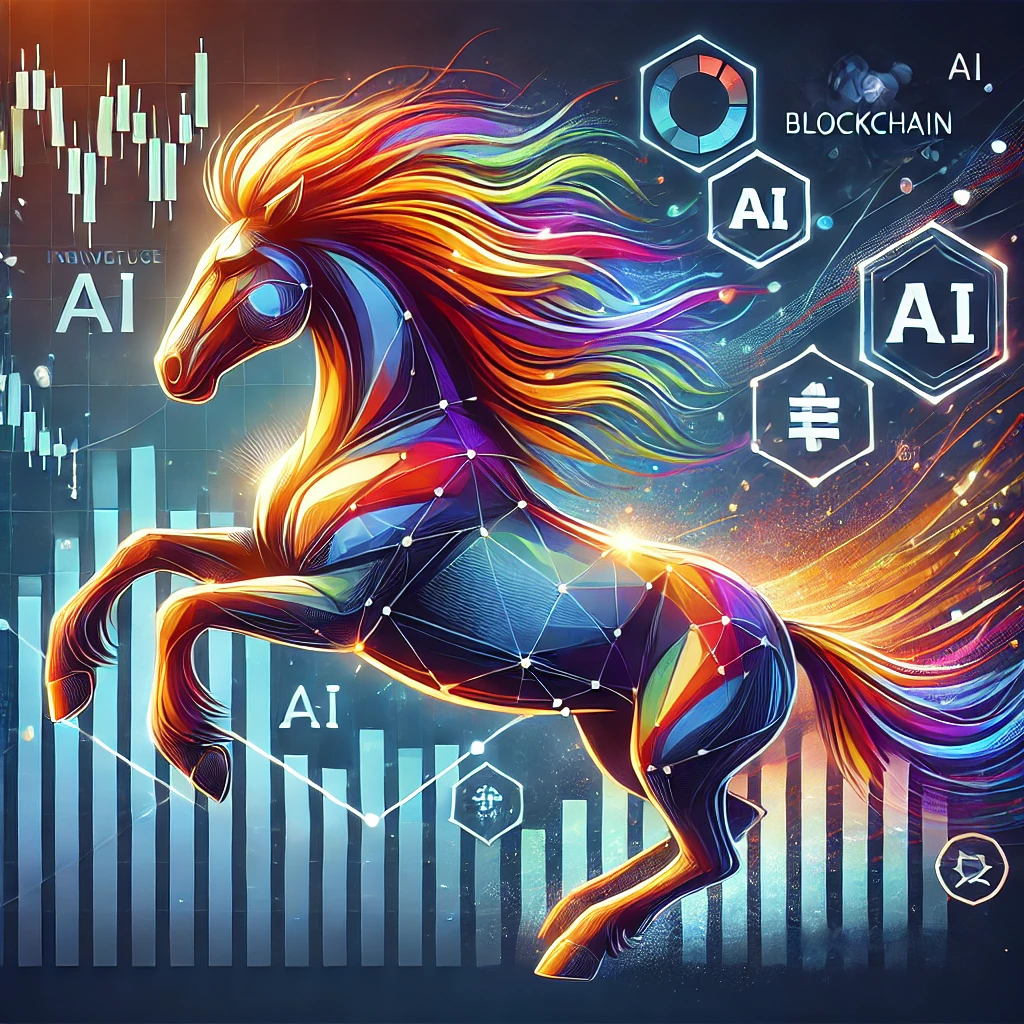
SYGNAL has leveraged the power of AI sentiment analysis to develop advanced trading strategies that optimize returns and manage risks effectively. Here are three notable examples:
US Renewable Energy Tech: This strategy tracks over 70 US-based companies in the renewable energy sector. It uses a Decarbonization Technology Score (DTS) to assess companies based on patent databases, industry publications, and scientific articles. The DTS is combined with short-term momentum signals to guide what and when to trade, aiming for high returns while managing volatility.
Fusion AI: This strategy combines traditional trading methodologies with advanced AI-driven sentiment analysis. By merging sentiment trends with price data and technical indicators, Fusion AI provides daily actionable insights, helping traders navigate the volatile crypto market. Its multidimensional approach ensures a comprehensive analysis, enhancing decision-making and optimizing returns.
AltcoinWave AI: Another innovative strategy by SYGNAL, AltcoinWave AI, leverages proprietary AI to manage top altcoins. This strategy integrates sentiment analysis and trend-following techniques, aiming to outperform Bitcoin and maximize traders' returns. By continuously analyzing market sentiment and adjusting strategies accordingly, AltcoinWave AI offers a dynamic and responsive approach to trading.
By integrating these advanced AI-driven strategies with blockchain verification, SYGNAL demonstrates its commitment to innovation, transparency, and effectiveness in crypto trading.
The Future of AI in Trading
Tomorrow's Trends Today
The integration of AI in trading is continually evolving, and several emerging trends are set to shape the future of AI sentiment trading models:
Advanced Sentiment Analysis Tools: Sentiment analysis tools are becoming more nuanced, allowing for a deeper understanding of market dynamics. These tools analyze vast amounts of data from news, social media, and other sources to gauge market sentiment and public opinion about finance. This enables traders to make more informed decisions by understanding the emotional drivers behind market trends.
Quantitative Market Psychology: Investment strategies increasingly incorporate quantitative market psychology and sentiment indicators. This approach provides a robust framework for anticipating and responding to market volatility, optimizing investment strategies in alignment with prevailing market sentiments. By employing technical and sentiment analysis tools, investors and analysts can gain deeper insights into market trends and investor behaviours.
Algorithmic Trading and AI Decision-Making: Many trading activities are driven by algorithms, underscoring the potential systemic risks arising from opaque AI decision-making processes. The future of trading is expected to involve a balanced approach that leverages the best technology while preserving and enhancing the role of human insight and accountability.
Regulatory Considerations: As AI continues to play a more significant role in trading, potential regulations around algorithmic trading practices and disclosures are being considered to ensure that investors are aware of AI's role in their investments. This is crucial for maintaining transparency and trust in AI-driven trading systems.
Integration of AI in Financial Planning and Forecasting: AI technologies are also integrated into financial planning and forecasting tools. These tools use machine learning to predict future trends based on historical data, providing traders with valuable insights that can inform their trading decisions.
The integration of advanced AI technologies into trading strategies is expected to grow, driven by AI's capabilities to analyze and interpret complex market data and sentiments. This will likely lead to more sophisticated and informed trading strategies that can adapt to and capitalize on market dynamics.
AI vs. Traditional Trading: A Showdown
Clash of the Titans
AI-based sentiment trading models and traditional quantitative trading strategies are two distinct approaches to financial markets, each with advantages and challenges. Here's a detailed comparison based on accuracy, scalability, and risk:
AI-Based Sentiment Trading Models
Pros | Cons |
---|---|
Accuracy AI-based sentiment trading models leverage natural language processing (NLP) to analyze vast amounts of unstructured data (e.g., news articles, social media posts) to gauge market sentiment. This can provide a more nuanced understanding of market dynamics that traditional models might miss. |
Risk Sentiment-based models can be susceptible to anomalies in data or sudden shifts in public opinion, which may not always correlate with fundamental market conditions, leading to potential volatility or misleading signals. |
Scalability These models can process and analyze data at a scale unmanageable for human traders, continuously updating predictions and strategies based on real-time data. |
Complexity and Interpretability The models can become "black boxes," where it's difficult to understand how decisions are made, complicating troubleshooting and trust in the system. |
Traditional Quantitative Trading Strategies
Pros | Cons |
---|---|
Stability and Predictability These strategies often rely on historical data and mathematical models that are well-understood and tested, providing a stable foundation for decision-making. |
Scalability While quantitative strategies can handle large datasets, they are typically less adept at processing the volume and variety of unstructured data that AI models can manage. |
Transparency Quantitative models typically use clearly defined rules and algorithms, making them easier to monitor, understand, and regulate. |
Adaptability Traditional models may not quickly adapt to sudden market changes as they rely heavily on historical data and predefined rules. This can be a disadvantage in highly volatile or rapidly evolving markets. |
Best of Both Worlds
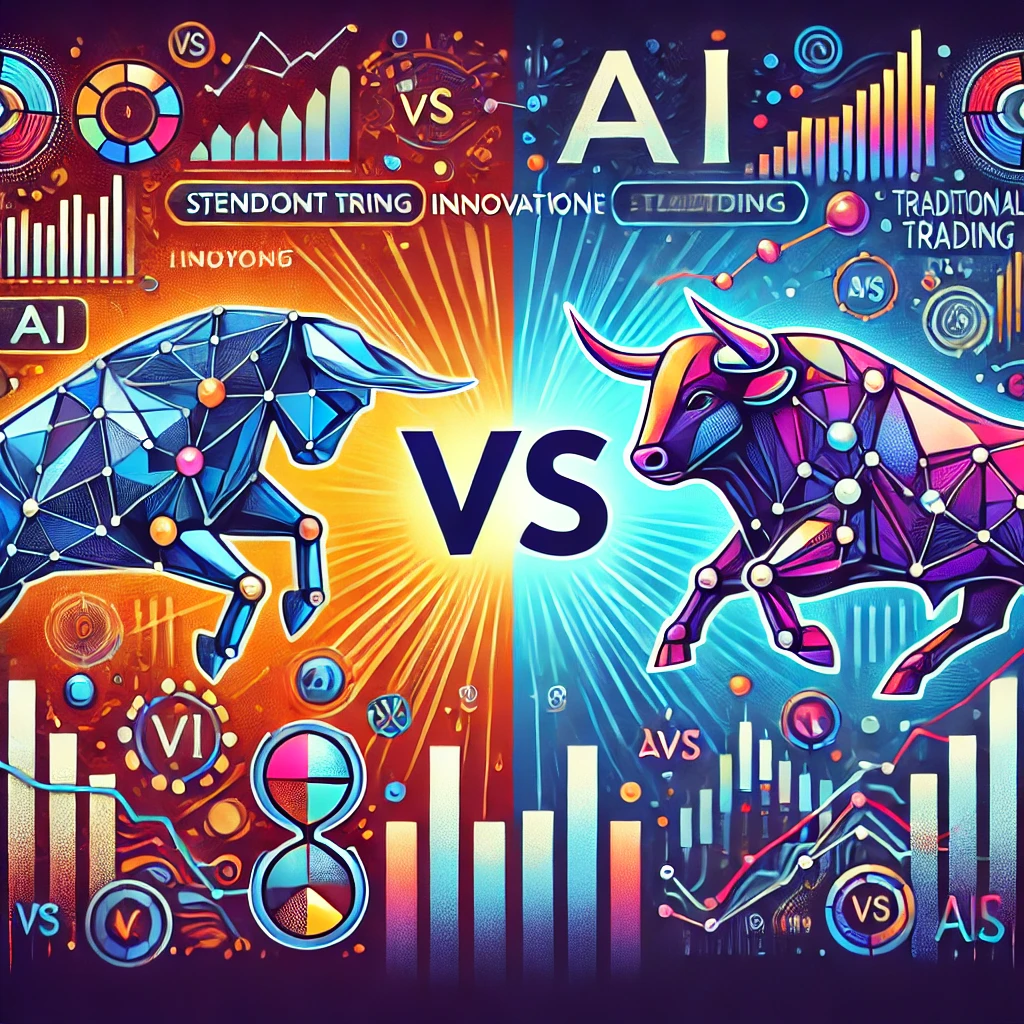
Combining AI-based sentiment trading models with traditional quantitative strategies can offer a balanced approach. This hybrid model leverages the strengths of both methods to enhance trading performance while mitigating associated risks. By integrating AI's real-time sentiment analysis with the stable, rule-based approach of quantitative models, traders can achieve more accurate predictions and robust risk management.
The Psychology of Markets
Mind Over Markets
AI-based sentiment trading models represent a significant evolution in applying technology to financial markets. They interact with traditional financial theories, such as the Behavioral Theory of Finance and the Animal Spirit Theory, in complementary and challenging ways.
AI Sentiment Trading Models and Market Psychology
Illustrating the Integration of AI Sentiment Analysis with Traditional Market Theories
AI Sentiment Trading Models
Behavioral Theory of Finance
Capture Emotional Drivers
Animal Spirit Theory
Recognizing Irrational Behaviors
Traditional Financial Theories
Challenging Rationality
Market Dynamics
Practical Implications
Alignment with Behavioral Theory of Finance: The Behavioral Theory of Finance suggests that psychological influences and biases affect the financial behaviours of investors and subsequent market outcomes. AI-based sentiment trading models align with this theory by analyzing vast amounts of data from news, social media, and other sources to gauge market sentiment. This capability allows these models to capture and quantify the emotional drivers of market trends, which are central to behavioural finance. For instance, these models can detect shifts in investor sentiment that might not be immediately obvious through traditional analysis, thereby providing a more nuanced understanding of market dynamics.
Challenge to Traditional Financial Theories: Traditional financial theories often assume rational behaviour among market participants. However, by focusing on sentiment and emotional drivers, AI-based models acknowledge and capitalize on irrational behaviours and psychological biases that can influence financial decisions. This challenges the rationality assumption underpinning many traditional theories, including the Efficient Market Hypothesis (EMH), which posits that asset prices fully reflect all available information.
Furthermore, the Behavioral Theory of Finance and the Animal Spirit Theory, which recognize the role of emotions and irrational behaviours in financial markets, can be seen as more aligned with the capabilities of AI-based sentiment models. By systematically analyzing emotional content, these models can provide insights that challenge the notion that markets are always driven by rational decisions.
Integration and Coexistence: While AI-based sentiment trading models challenge some aspects of traditional financial theories by introducing a systematic way to analyze emotions and biases, they also help validate and operationalize these theories. By providing empirical data on how sentiments and non-rational behaviours affect market prices, AI models can enhance the applicability of behavioural finance theories in real-world trading scenarios.
These models contribute to a more comprehensive understanding of market dynamics by integrating traditional financial analysis with insights into behavioural biases and sentiment-driven market movements. This integration can lead to more robust trading strategies that account for both rational financial fundamentals and market behaviour's less rational, sentiment-driven aspects.
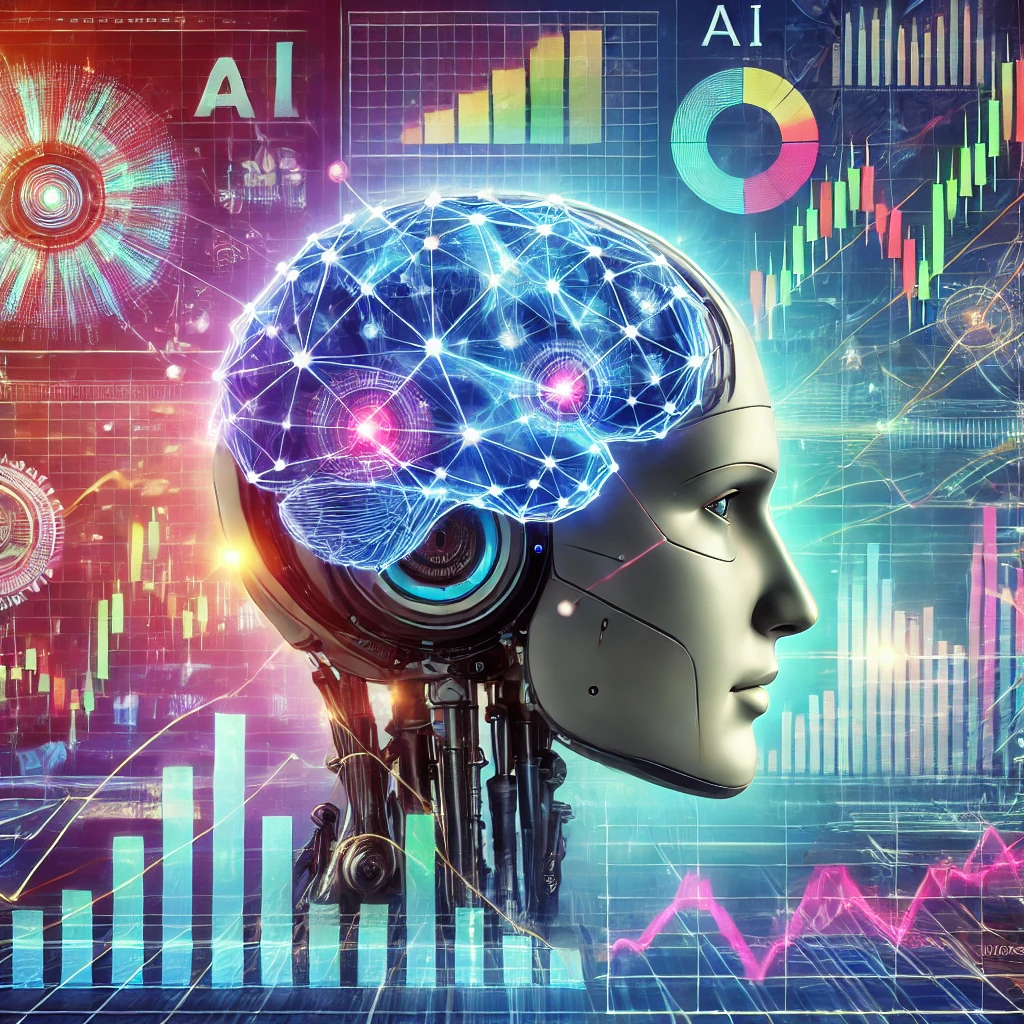
Brains and Bots
In summary, AI-based sentiment trading models align with and challenge traditional financial theories by providing tools to measure and act upon market behaviour's emotional and psychological elements, which have been underappreciated in more conventional economic theories. This dual role helps advance the field of finance towards a more integrated approach that considers both rational and irrational market forces.
Wrapping It Up
AI sentiment trading models are revolutionizing the trading landscape by providing sophisticated tools that analyze market sentiment through NLP, AI, and ML. These models offer traders a competitive edge by turning raw data into actionable insights, allowing for more informed and timely trading decisions.
These models enhance predictive accuracy and offer a deeper understanding of market dynamics by integrating sentiment analysis with traditional trading strategies. However, traders must navigate challenges such as handling ambiguous data and rapid sentiment shifts and be mindful of ethical and regulatory considerations.
SYGNAL's advanced AI-driven strategies, such as Fusion AI, AltcoinWave AI, and US Renewable Energy Tech, exemplify the power of combining sentiment analysis with blockchain-based auditing to ensure transparency and reliability. As AI technologies evolve, their integration into trading strategies promises more sophisticated and informed approaches to capitalizing on market dynamics.